Our Global Health Initiative launched in 2016 with the goal of eliminating pediatric health disparities around the world. We aim to address the most pressing pediatric health issues through better care for medically underserved populations. This leadership helps us achieve our mission of caring for all children. A broad range of education and research projects improves health outcomes. They also offer enriching opportunities for experienced faculty and emerging leaders to advance clinical excellence.
Healing hearts in Uganda
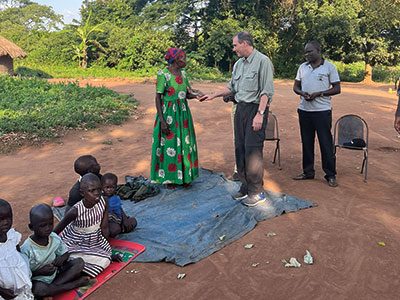
Dr. Craig Sable and team train partners in Uganda.
Craig Sable, M.D., interim chief of Cardiology, improves care for young people with rheumatic heart disease (RHD) in Uganda. Donors, including the Karp Family Foundation, Huron Philanthropies, Zachary Blumenfeld Fund and the Wood family, make this possible. RHD affects 50 million people, mostly children, worldwide. It claims 400,000 lives each year.
Dr. Sable and Ugandan partners completed important research showing that early RHD detection, coupled with monthly penicillin treatment, can protect the heart. They are working on practical solutions, such as a new portable device with artificial intelligence (AI) that can easily screen for RHD.
In 2023, Dr. Sable led two missions in Uganda where he and his team did surgeries and special tests for 18 children with RHD. They also taught local doctors new skills to help more kids on their own.
Plastic surgery and reconstructive care in Kenya and Nepal
Each year our Craniofacial & Pediatric Plastic Surgery team, under the leadership of Johnston Family Professor of Pediatric Plastic Surgery and Chief of Pediatric Plastic Surgery Gary Rogers, M.D., J.D., LL.M., M.B.A., M.P.H., provides opportunities for fellows to participate in surgical missions.
In 2024, Perry Bradford, M.D., traveled to the Moi Teaching Hospital in Eldoret, Kenya where she provided patients with burn, pressure wound and cleft reconstruction. She built community connections with the local plastic surgeons and educated registrars and medical students. “This gave me firsthand experience working in a community with limited resources and forced me to be more creative,” Dr. Bradford says. “The experience inspired me to examine what it means to have consistent access to advanced tools and equipment.”
In 2022, a group traveled to Nepal to provide care. Some patients arrived after days of travel by yak or buffalo. One child with a burn injury recovered use of her hand. The team educated local providers to deliver life-changing treatments unavailable in Nepal.
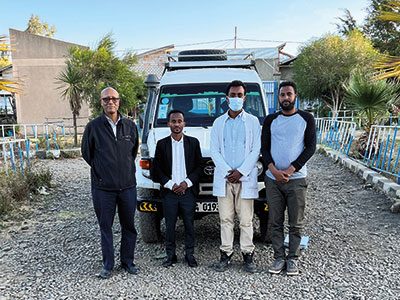
Dr. Tesfaye Zelleke, left, and team in Ethopia.
Elevating epilepsy care in Ethiopia
Neurologist Tesfaye Zelleke, M.D., and partners in Ethiopia are seeking to improve the lives of children with epilepsy. The BAND Foundation provides support. Ethiopia has a population of about 120 million yet only a handful of pediatric neurologists.
Dr. Zelleke’s team trained nonspecialist providers to diagnose and treat children in the primary care setting. They also launched a mobile epilepsy clinic to provide community care and build the capacity of local clinicians. In collaboration with advocacy groups, the team educates the public about epilepsy with a goal of reducing stigma.
New hope in Norway
In 2023, our Division of Colorectal & Pelvic Reconstruction shared its expertise with clinicians at Oslo University Hospital, Rikshospitalet, in Norway. This effort was a key first step in Oslo becoming the first dedicated colorectal center in Scandinavia.
Marc Levitt, M.D., and team members performed complex surgeries otherwise unavailable for waiting patients. They led an academic conference. They held clinics to educate nurses, reviewed patient records and made care recommendations. Specialized care enabled a young patient with significant bowel difficulties to recover function and lead a normal life.
The team will travel to South Africa, the Czech Republic and Spain in 2024. Donors, including The Dune Road Foundation and Deanna and Howard Bayless, make this work possible.
Improving outcomes for babies in the Congo
AI can be a valuable tool for diagnosing genetic conditions. It detects unique facial patterns that clinicians without genetics training can miss. However, existing facial analysis software struggles in nonwhite populations.
A team led by Marius George Linguraru, D.Phil., M.A., M.Sc., the Connor Family Professor of Research and Innovation and principal investigator in the Sheikh Zayed Institute for Pediatric Surgical Innovation, is working to improve the newborn diagnosis rate worldwide. They are testing smartphone software in the Democratic Republic of Congo. Diverse newborn data improves AI’s ability to detect a variety of genetic conditions in more children. Early detection, diagnosis and informed care lead to better health outcomes.
Nephrology care for kids in Jamaica
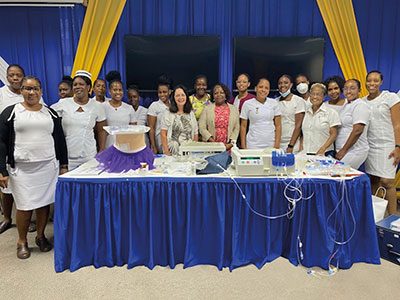
Jennifer Carver and Dr. Marva Moxey-Mims, center, with staff at Bustamante Children’s Hospital.
Marva Moxey-Mims, M.D., chief of Nephrology, is bringing care to children with kidney disease in Jamaica, with a goal of improving health equity. An International Pediatric Nephrology Association grant helped make it possible.
On a recent trip, Dr. Moxey-Mims and a small team — including Jennifer Carver, RN, CNN, lead peritoneal dialysis nurse at Children’s National, and three pediatric nephrologists from Jamaica — trained nearly 30 nurses from Jamaican hospitals. Nurses received hands-on dialysis education to improve their clinical skills. The team also worked to educate the community in disease awareness and prevention.
Read more stories like this one in the latest issue of Believe magazine.