New datasets predict surgeon performance during complications
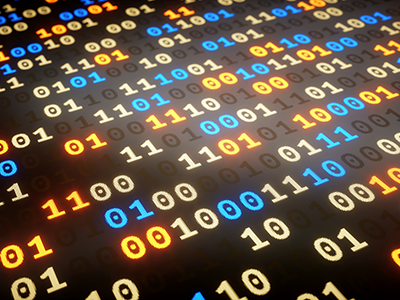
In a new study published in JAMA Network Open, experts at Children’s National and allied institutions created and validated the first dataset to depict hemorrhage control for machine learning applications, the simulated outcomes following carotid artery laceration (SOCAL) video dataset.
Computer algorithms, such as machine learning and computer vision, are increasingly able to discover patterns in visual data, powering exciting new technologies worldwide. At Children’s National Hospital, physician-scientists develop and apply these advanced algorithms to make surgery safer by studying surgical video.
The big picture
In a new study published in JAMA Network Open, experts at Children’s National and allied institutions created and validated the first dataset to depict hemorrhage control for machine learning applications, the simulated outcomes following carotid artery laceration (SOCAL) video dataset.
The authors designed SOCAL to serve as a benchmark for data-science applications, including object detection, performance metric development and outcome prediction. Hemorrhage control is a high-stakes adverse event that can pose unique challenges for video analysis. With SOCAL, the authors aim to solve a valuable use case with algorithms.
“As neurosurgeons, we are often called to perform high-risk and high-impact procedures. No one is more passionate about making surgery safer,” said Daniel Donoho, M.D., neurosurgeon at Children’s National Hospital and senior author of the study. “Our team at Children’s National and the Sheikh Zayed Institute is poised to lead this exciting new field of surgical data science.”
The hold-up in the field
These algorithms require raw data for their development, but the field lacks datasets that depict surgeons managing complications.
By creating automated insights from surgical video, these tools may one day improve patient care by detecting complications before patients are harmed, facilitating surgeon development.
Why it matters
“Until very recently, surgeons have not known what may be possible with large quantities of surgical video captured each day in the operating room,” said Gabriel Zada, M.D., M.S., F.A.A.N.S., F.A.C.S., director of the Brain Tumor Center at the University of Southern California (USC) and co-author of the study. “Our team’s research led by Dr. Donoho shows the feasibility and the potential of computer vision analysis in surgical skill assessment, virtual coaching and simulation training of surgeons.”
The lack of videos of adverse events creates a dataset bias which hampers surgical data science. SOCAL was designed to meet this need. After creating a cadaveric simulator of internal carotid artery injury and training hundreds of surgeons on the model at nationwide courses, the authors then developed computational models to measure and improve performance.
“We are currently comparing our algorithms to experts, including those developed using the SOCAL dataset,” Dr. Donoho said. “Human versus machine, and our patients are ultimately the winners in the competition.”
What’s next
The authors are also building a nationwide collective of surgeons and data scientists to share data and improve algorithm performance through exciting partnerships with USC, California Institute of Technology and other institutions.
You can read the full study “Utility of the Simulated Outcomes Following Carotid Artery Laceration Video Data Set for Machine Learning Applications” in JAMA Network Open.