Tag Archive for: Marius George Linguraru
Global expert consensus defines first framework for building trustworthy AI in health care
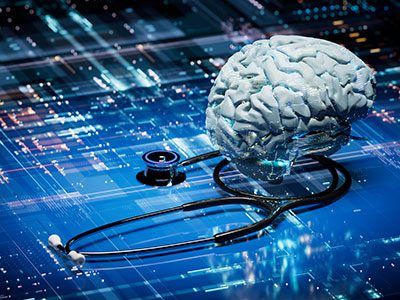
The guidelines are the first globally acknowledged framework for developing and deploying health care AI applications and gauging whether the information they generate can be trusted or not.
More than 100 international experts in the application of artificial intelligence (AI) in health care published the first set of consensus guidelines that outline criteria for what it means for an AI tool to be considered trustworthy when implemented in health care settings.
The guidelines, published in the journal the BMJ, are the first globally acknowledged framework for developing and deploying health care AI applications and gauging whether the information they generate can be trusted or not.
What this means
Called the FUTURE-AI framework, the consensus guidelines are organized based on six guiding principles:
- Fairness
- Universality
- Traceability
- Usability
- Robustness
- Explainability
The cadre of experts reviewed and agreed upon a set of 30 best practices that fall within the six larger categories. These practices address technical, clinical, socio-ethical and legal aspects of trustworthy AI. The recommendations cover the entire lifecycle of health care AI: design, development and validation, regulation, deployment and monitoring.
The authors encourage researchers and developers to take these recommendations into account in the proof-of-concept phase for AI-driven applications to facilitate future translation to clinical practice.
Why it matters
“Patients, clinicians, health organizations and authorities need to know that information and analysis generated by AI can be trusted, or these tools will never make the leap from theoretical to real world application in a clinical setting,” says Marius George Linguraru, DPhil, MA, MSc, Connor Family Professor for Research and Innovation in the Sheikh Zayed Institute for Surgical Innovation at Children’s National Hospital and co-author of the guidelines. “Bringing so many international and multi-disciplinary perspectives together to outline the characteristics of a trustworthy medical AI application is part of what makes this work unique. It is my hope that finding such broad consensus will shed light on the greater good AI can bring to clinics and help us avoid problems before they ever impact patients.”
The FUTURE-AI consortium was founded by Karim Lekadir, PhD, ICREA Research Professor at the University of Barcelona in 2021 and now comprises 117 interdisciplinary experts from 50 countries representing all continents, including AI scientists, clinical researchers, biomedical ethicists and social scientists. Over a 2-year period, the consortium established these guiding principles and best practices for trustworthy and deployable AI through an iterative process comprising an in-depth literature review, a modified Delphi survey and online consensus meetings. Dr. Linguraru contributed with a unique perspective on AI for pediatric care and rare diseases.
What’s next
The authors note that, “progressive development and adoption of medical AI tools will lead to new requirements, challenges and opportunities. For some of the recommendations, no clear standard on how these should be addressed yet exists.”
To tackle this uncertainty, they propose FUTURE-AI as a dynamic, living framework. This includes a dedicated website to allow the global community to participate in the FUTURE-AI network. Visitors can provide feedback based on their own experiences and perspectives. The input gathered will allow the consortium to refine the FUTURE-AI guidelines and learn from other voices.
Read the full manuscript outlining all 30 best practices: FUTURE-AI: international consensus guideline for trustworthy and deployable artificial intelligence in healthcare
Marius George Linguraru appointed as president of the MICCAI Society
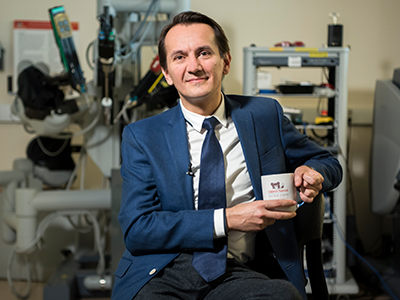
“MICCAI has been a professional home for me throughout my career and I am deeply honored to have a chance to give back to the organization,” said Dr. Linguraru.
We’re pleased to announce that Marius George Linguraru, DPhil, MA, MSc, Connor Family professor and endowed chair in Research and Innovation at Children’s National, has been elected as president of the Medical Image Computing and Computer Assisted Intervention (MICCAI) Society board of directors. Dr. Linguraru has been involved in the society since he attended his first MICCAI conference in 2001. He was elected to the board of directors in 2021 and chairs the Career Development Working Group. Dr. Linguraru was instrumental in establishing the MICCAI Mentorship Program, the MICCAI Start-up Village and the AFRICAI Special Interest Group. He also served as the program chair of MICCAI 2024, which received and reviewed a record number of paper submissions. He will begin his three-year term as president on February 1, 2025. Watch Dr. Linguraru’s brief inaugural message to members here.
“MICCAI has been a professional home for me throughout my career and I am deeply honored to have a chance to give back to the organization,” said Dr. Linguraru. “I believe the society is poised not just to meet the challenges of the next few years, but to thrive as an essential leadership forum for the growth of medical image computing, computer assisted intervention and artificial intelligence in healthcare.”
Dr. Linguraru leads the AI research initiatives at Children’s National and serves as principal investigator in the Sheikh Zayed Institute for Pediatric Surgical Innovation. His award-winning team builds artificial intelligence applications to expand health equity and access to pediatric healthcare when diseases are rare and resources are limited. Through partnerships between Children’s National and Virginia Tech and Microsoft, Dr. Linguraru also plays an integral role in exploring how generative AI can improve pediatric care.
Meanwhile, Caroline Essert, PhD, MSc, completes her term as president of the MICCAI Society on January 31, 2025.
“It has been an honor to contribute to the growth and vibrancy of this incredible community,” said Dr. Essert. “I extend my warmest welcome to Dr. Linguraru as the incoming president of the MICCAI Board. I am confident that under his leadership, the MICCAI Society will reach new heights and continue to serve as a beacon of excellence in our field.” Read her full farewell message here.
To learn more about the MICCAI Society, click here.
AI’s transformative potential in radiology
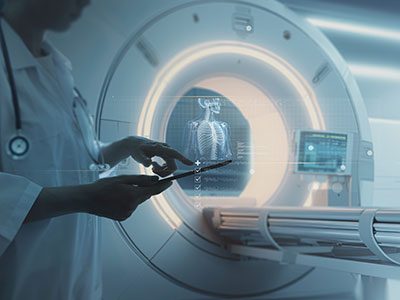
The adoption of artificial intelligence (AI) has the potential to enhance radiological imaging, improve diagnostic capabilities and reduce burnout in the field.
The adoption of artificial intelligence (AI) has the potential to enhance radiological imaging, improve diagnostic capabilities and reduce burnout in the field, provided that physicians and scientists work together to ensure its careful integration into the practice of medicine, according to a special report in Radiology: Artificial Intelligence, a journal of the Radiological Society of North America (RSNA).
Assembled by experts in radiology, medical imaging and machine learning, the special report lays out the clinical, cultural, computation and regulatory considerations that are being introduced, particularly as generative AI models become part of the field.
“AI tools can play a key role in radiology, but radiologists must be able to trust in the systems’ design and receive adequate training. As the physicians most familiar with these tools, radiologists should establish clear guidelines regarding clinical accountability,” said Marius George Linguraru, D.Phil., M.A., M.Sc., the Connor Family Professor in Research and Innovation and principal investigator in the Sheikh Zayed Institute for Pediatric Surgical Innovation.
Moving the field forward
Dr. Linguraru and his peers assembled the report based on a series of seminars hosted by RSNA and the Medical Image Computing and Computer Assisted Intervention (MICCAI) Society. They collected input from multidisciplinary experts to outline the current clinical uses of AI and its future potential.
The experts agreed that collaboration between radiologists and AI scientists will be essential to successfully integrate AI into the discipline of radiology. This partnership should focus on establishing a unified agenda, shared language and clear expectations of the tools developed. By working together, they can ensure that AI tools are designed and implemented to meet the practical needs of radiology, particularly with the incorporation of language and vision models.
What’s next
Among the challenges ahead, clinical institutions must align their staffing, data management and computational resources to deploy and monitor AI systems effectively. This alignment includes ensuring that personnel are adequately trained to use AI tools, that data is managed and processed efficiently and that sufficient computational power is available to support AI operations. Cloud computing may be vital to hospitals that don’t have hardware and technical maintenance resources.
“The successful integration of AI in radiology depends on trust in AI design, collaborative efforts between radiologists and AI scientists, and the alignment of clinical resources to support AI deployment,” Dr. Linguraru said. “With these factors in place, AI can play a transformative role in improving radiological practices and outcomes.”
Read the special report “Clinical, Cultural, Computational, and Regulatory Considerations to Deploy AI in Radiology: Perspectives of RSNA and MICCAI Experts” in Radiology: Artificial Intelligence.
Around the world
Our Global Health Initiative launched in 2016 with the goal of eliminating pediatric health disparities around the world. We aim to address the most pressing pediatric health issues through better care for medically underserved populations. This leadership helps us achieve our mission of caring for all children. A broad range of education and research projects improves health outcomes. They also offer enriching opportunities for experienced faculty and emerging leaders to advance clinical excellence.
Healing hearts in Uganda
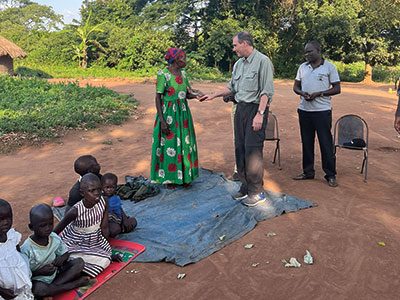
Dr. Craig Sable and team train partners in Uganda.
Craig Sable, M.D., interim chief of Cardiology, improves care for young people with rheumatic heart disease (RHD) in Uganda. Donors, including the Karp Family Foundation, Huron Philanthropies, Zachary Blumenfeld Fund and the Wood family, make this possible. RHD affects 50 million people, mostly children, worldwide. It claims 400,000 lives each year.
Dr. Sable and Ugandan partners completed important research showing that early RHD detection, coupled with monthly penicillin treatment, can protect the heart. They are working on practical solutions, such as a new portable device with artificial intelligence (AI) that can easily screen for RHD.
In 2023, Dr. Sable led two missions in Uganda where he and his team did surgeries and special tests for 18 children with RHD. They also taught local doctors new skills to help more kids on their own.
Plastic surgery and reconstructive care in Kenya and Nepal
Each year our Craniofacial & Pediatric Plastic Surgery team, under the leadership of Johnston Family Professor of Pediatric Plastic Surgery and Chief of Pediatric Plastic Surgery Gary Rogers, M.D., J.D., LL.M., M.B.A., M.P.H., provides opportunities for fellows to participate in surgical missions.
In 2024, Perry Bradford, M.D., traveled to the Moi Teaching Hospital in Eldoret, Kenya where she provided patients with burn, pressure wound and cleft reconstruction. She built community connections with the local plastic surgeons and educated registrars and medical students. “This gave me firsthand experience working in a community with limited resources and forced me to be more creative,” Dr. Bradford says. “The experience inspired me to examine what it means to have consistent access to advanced tools and equipment.”
In 2022, a group traveled to Nepal to provide care. Some patients arrived after days of travel by yak or buffalo. One child with a burn injury recovered use of her hand. The team educated local providers to deliver life-changing treatments unavailable in Nepal.
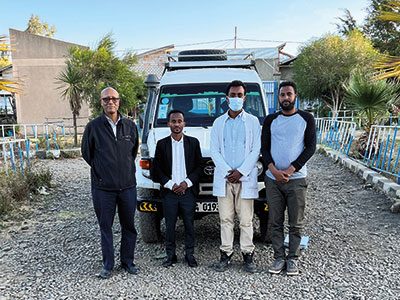
Dr. Tesfaye Zelleke, left, and team in Ethopia.
Elevating epilepsy care in Ethiopia
Neurologist Tesfaye Zelleke, M.D., and partners in Ethiopia are seeking to improve the lives of children with epilepsy. The BAND Foundation provides support. Ethiopia has a population of about 120 million yet only a handful of pediatric neurologists.
Dr. Zelleke’s team trained nonspecialist providers to diagnose and treat children in the primary care setting. They also launched a mobile epilepsy clinic to provide community care and build the capacity of local clinicians. In collaboration with advocacy groups, the team educates the public about epilepsy with a goal of reducing stigma.
New hope in Norway
In 2023, our Division of Colorectal & Pelvic Reconstruction shared its expertise with clinicians at Oslo University Hospital, Rikshospitalet, in Norway. This effort was a key first step in Oslo becoming the first dedicated colorectal center in Scandinavia.
Marc Levitt, M.D., and team members performed complex surgeries otherwise unavailable for waiting patients. They led an academic conference. They held clinics to educate nurses, reviewed patient records and made care recommendations. Specialized care enabled a young patient with significant bowel difficulties to recover function and lead a normal life.
The team will travel to South Africa, the Czech Republic and Spain in 2024. Donors, including The Dune Road Foundation and Deanna and Howard Bayless, make this work possible.
Improving outcomes for babies in the Congo
AI can be a valuable tool for diagnosing genetic conditions. It detects unique facial patterns that clinicians without genetics training can miss. However, existing facial analysis software struggles in nonwhite populations.
A team led by Marius George Linguraru, D.Phil., M.A., M.Sc., the Connor Family Professor of Research and Innovation and principal investigator in the Sheikh Zayed Institute for Pediatric Surgical Innovation, is working to improve the newborn diagnosis rate worldwide. They are testing smartphone software in the Democratic Republic of Congo. Diverse newborn data improves AI’s ability to detect a variety of genetic conditions in more children. Early detection, diagnosis and informed care lead to better health outcomes.
Nephrology care for kids in Jamaica
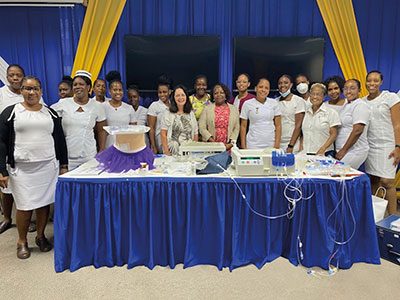
Jennifer Carver and Dr. Marva Moxey-Mims, center, with staff at Bustamante Children’s Hospital.
Marva Moxey-Mims, M.D., chief of Nephrology, is bringing care to children with kidney disease in Jamaica, with a goal of improving health equity. An International Pediatric Nephrology Association grant helped make it possible.
On a recent trip, Dr. Moxey-Mims and a small team — including Jennifer Carver, RN, CNN, lead peritoneal dialysis nurse at Children’s National, and three pediatric nephrologists from Jamaica — trained nearly 30 nurses from Jamaican hospitals. Nurses received hands-on dialysis education to improve their clinical skills. The team also worked to educate the community in disease awareness and prevention.
Read more stories like this one in the latest issue of Believe magazine.
Artificial – and accelerated – intelligence: endless applications to expand health equity
In the complex world of pediatric diseases, researchers need access to data to develop clinical trials and the participation of vulnerable patients to develop new devices and therapies. Both are in short supply, given that most children are born healthy, and most severe pediatric diseases are rare.
That creates a dilemma: how do researchers build a foundation to advance new treatments? Enter artificial intelligence (AI).
“AI is the equalizer: accelerated intelligence for sick kids. No other advance on the horizon holds more promise for improving equity and access to pediatric healthcare when diseases are rare and resources are limited,” says Marius George Linguraru, D.Phil., M.A., M.Sc., the Connor Family Professor in Research and Innovation and principal investigator in the Sheikh Zayed Institute for Pediatric Surgical Innovation (SZI). “AI will shrink the distance between patient and provider, allowing our physicians and scientists to provide targeted healthcare for children more efficiently. The possibilities are endless.”
Why we’re excited
By pioneering AI innovation programs at Children’s National Hospital, Dr. Linguraru and the AI experts he leads are ensuring patients and families benefit from a coming wave of technological advances. The team is teaching AI to interpret complex data that could otherwise overwhelm clinicians. Their work will create systems to identify at-risk patients, forecast disease and treatment patterns, and support complex clinical decisions to optimize patient care and hospital resources. Already, the AI team at SZI has developed data-driven tools touching nearly every corner of the hospital:
- AI for rheumatic heart disease (RHD): In partnership with Children’s National cardiology leaders, including Craig Sable, M.D., the Uganda Heart Institute and Cincinnati Children’s Hospital, the AI team has developed an algorithm that can use low-cost, portable ultrasound imaging to detect RHD in children and young adults, a disease that takes nearly 400,000 lives annually in limited-resource countries. Early testing shows the AI platform has the same accuracy as a cardiologist in detecting RHD, paving the way for earlier treatment with life-saving antibiotics. This year, Children’s National physicians will be in Uganda, screening 200,000 children with local cardiology experts and AI technology.
- Newborn screening for genetic conditions with mGene: Working with Rare Disease Institute clinicians and Chief of Genetics and Metabolism Debra Regier, M.D., the AI team has built technology to detect rare genetic disorders, using an algorithm and a smartphone camera to identify subtle changes in facial features. Tested on patients from over 30 countries and published in The Lancet Digital Health, the application helps screen children for advanced care when a geneticist may not be within reach. With funding from the National Institutes of Health, Children’s National and its research partners are piloting a newborn screening program in the Democratic Republic of the Congo.
- Pediatric brain tumors: To improve and personalize the treatment decisions for children with brain tumors, Dr. Linguraru’s team is working with Brain Tumor Institute Director Roger Packer, M.D., the Gilbert Family Distinguished Professor of Neurofibromatosis, on algorithms that can characterize and measure brain tumors with unprecedented precision. The team recently won the International Pediatric Brain Tumor Segmentation Challenge, distinguishing the Children’s National algorithm as among the best in the world.
- Ultra-low field magnetic resonance imaging (MRI): With a grant from the Bill & Melinda Gates Foundation, the AI team is working alongside Children’s Hospital Los Angeles, King’s College London and the UNITY Consortium to expand global brain imaging capacity. The consortium is helping clinicians in limited-resource countries improve the treatment of neonatal neurological conditions, using AI to boost the quality of ultra-low field MRI and expand access to this portable and more affordable imaging option.
- Federated learning: Children’s National has collaborated with NVIDIA and other industry leaders to accelerate AI advances through federated learning. Under this approach, institutions share AI models rather than data, allowing them to collaborate without exposing patient information or being constrained by essential data-sharing restrictions. The SZI team was the only pediatric partner invited to join the largest federated learning project of its kind, studying the lungs of COVID-19 patients. Details were published in Nature Medicine.
Children’s National leads the way
Looking ahead, the Children’s National AI team is pursuing a wide range of advances in clinical care. To support patients treated at multiple clinics, they are developing systems to harmonize images from different scanners and protocols, such as MRI machines made by different manufacturers. Similar work is underway to analyze pathology samples from different institutions consistently.
Automation is also making care more efficient. For example, using data from 1 million chest X-rays, the team is collaborating with NVIDIA to develop a conversational digital assistant that will allow physicians to think through 14 possible diagnoses.
Dr. Linguraru says he and his colleagues are galvanized by the jarring statistic that one in three children with a rare disease dies before age 5. While well-implemented AI initiatives can change outcomes, he says the work must be done thoughtfully.
“In the future, patients will be evaluated by human clinicians and machines with extraordinary powers to diagnose illness and determine treatments,” Dr. Linguraru said. “Our team at Children’s National is leading conversations about the future of pediatric healthcare with a focus on safety, resource allocation and basic equity.”
Learn more about our AI initiatives
Innovation leaders at Children’s National Hospital are building a community of AI caregivers through educational and community-building events. At the inaugural Symposium on Artificial Intelligence in 2023 at the Children’s National Research & Innovation Campus, experts from Virginia Tech, JLABS, Food and Drug Administration, Pfizer, Oracle Health, NVIDIA, AWS Health and elsewhere laid out a vision for using data to advance pediatric medicine. The symposium will return on Sept. 6.
Dr. Linguraru is the program chair of MICCAI 2024, the top international meeting on medical image computing and computer-assisted intervention and the preeminent forum for disseminating AI developments in healthcare. The conference is an educational platform for scientists and clinicians dedicated to AI in medical imaging, with a focus on global health equity. It will take place for the first time in Africa on Oct. 6-10.
Novel AI platform matches cardiologists in detecting rheumatic heart disease
Artificial intelligence (AI) has the potential to detect rheumatic heart disease (RHD) with the same accuracy as a cardiologist, according to new research demonstrating how sophisticated deep learning technology can be applied to this disease of inequity. The work could prevent hundreds of thousands of unnecessary deaths around the world annually.
Developed at Children’s National Hospital and detailed in the latest edition of the Journal of the American Heart Association, the new AI system combines the power of novel ultrasound probes with portable electronic devices installed with algorithms capable of diagnosing RHD on echocardiogram. Distributing these devices could allow healthcare workers, without specialized medical degrees, to carry technology that could detect RHD in regions where it remains endemic.
RHD is caused by the body’s reaction to repeated Strep A bacterial infections and can cause permanent heart damage. If detected early, the condition is treatable with penicillin, a widely available antibiotic. In the United States and other high-income nations, RHD has been almost entirely eradicated. However, in low- and middle-income countries, it impacts the lives of 40 million people, causing nearly 400,000 deaths a year.
“This technology has the potential to extend the reach of a cardiologist to anywhere in the world,” said Kelsey Brown, M.D., a cardiology fellow at Children’s National and co-lead author on the manuscript with Staff Scientist Pooneh Roshanitabrizi, Ph.D. “In one minute, anyone trained to use our system can screen a child to find out if their heart is demonstrating signs of RHD. This will lead them to more specialized care and a simple antibiotic to prevent this degenerative disease from critically damaging their hearts.”
The big picture
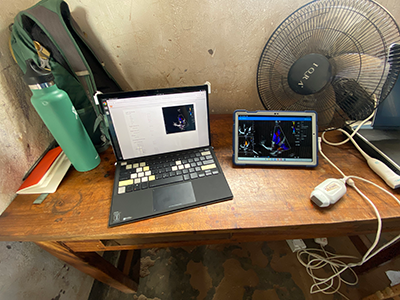
The new AI system combines the power of novel ultrasound probes with portable electronic devices installed with algorithms capable of diagnosing RHD on echocardiogram.
Millions of citizens in impoverished countries have limited access to specialized care. Yet the gold standard for diagnosing RHD requires a highly trained cardiologist to read an echocardiogram — a non-invasive and widely distributed ultrasound imaging technology. Without access to a cardiologist, the condition may remain undetected and lead to complications, including advanced cardiac disease and even death.
According to the new research, the AI algorithm developed at Children’s National identified mitral regurgitation in up to 90% of children with RHD. This tell-tale sign of the disease causes the mitral valve flaps to close improperly, leading to backward blood flow in the heart.
Beginning in March, Craig Sable, M.D., interim division chief of Cardiology, and his partners on the project will implement a pilot program in Uganda incorporating AI into the echo screening process of children being checked for RHD. The team believes that a handheld ultrasound probe, a tablet and a laptop — installed with the sophisticated, new algorithm — could make all the difference in diagnosing these children early enough to change outcomes.
“One of the most effective ways to prevent rheumatic heart disease is to find the patients that are affected in the very early stages, give them monthly penicillin for pennies a day and prevent them from becoming one of the 400,000 people a year who die from this disease,” Dr. Sable said. “Once this technology is built and distributed at a scale to address the need, we are optimistic that it holds great promise to bring highly accurate care to economically disadvantaged countries and help eradicate RHD around the world.”
Children’s National Hospital leads the way
To devise the best approach, two Children’s National experts in AI — Dr. Roshanitabrizi and Marius George Linguraru, D.Phil., M.A., M.Sc., the Connor Family Professor in Research and Innovation and principal investigator in the Sheikh Zayed Institute for Pediatric Surgical Innovation — tested a variety of modalities in machine learning, which mimics human intelligence, and deep learning, which goes beyond the human capacity to learn. They combined the power of both approaches to optimize the novel algorithm, which is trained to interpret ultrasound images of the heart to detect RHD.
Already, the AI algorithm has analyzed 39 features of hearts with RHD that cardiologists cannot detect or measure with the naked eye. For example, cardiologists know that the heart’s size matters when diagnosing RHD. Current guidelines lay out diagnostic criteria using two weight categories — above or below 66 pounds — as a surrogate measure for the heart’s size. Yet the size of a child’s heart can vary widely in those two groupings.
“Our algorithm can see and make adjustments for the heart’s size as a continuously fluid variable,” Dr. Roshanitabrizi said. “In the hands of healthcare workers, we expect the technology to amplify human capabilities to make calculations far more quickly and precisely than the human eye and brain, saving countless lives.”
Among other challenges, the team had to design new ways to teach the AI to handle the inherent clinical differences found in ultrasound images, along with the complexities of evaluating color Doppler echocardiograms, which historically have required specialized human skill to evaluate.
“There is a true art to interpreting this kind of information, but we now know how to teach a machine to learn faster and possibly better than the human eye and brain,” Dr. Linguraru said. “Although we have been using this diagnostic and treatment approach since World War II, we haven’t been able to share this competency globally with low- and middle-income countries, where there are far fewer cardiologists. With the power of AI, we expect that we can, which will improve equity in medicine around the world.”
Federated learning: A solution to AI’s data-sharing challenges
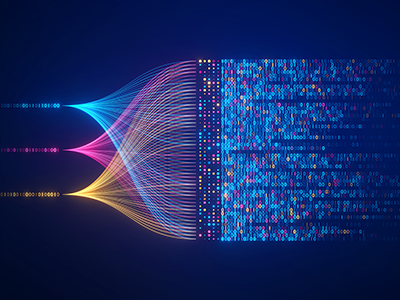
Federated learning can solve data-sharing challenges, allowing nimble collaboration across institutions to drive medical advances using artificial intelligence (AI).
Federated learning can solve data-sharing challenges, allowing nimble collaboration across institutions to drive medical advances using artificial intelligence (AI), according to a new manuscript from 10 thought leaders in AI and machine learning in medicine.
In Health Informatics Journal, these leading experts on how technology is shaping medicine shared a conversation that they had at the Radiology Society of North America’s conference. They weighed challenges facing AI, including barriers to data sharing because of privacy rules that prevent the distribution of information to different institutions. With federated learning, models are shared – rather than data – allowing institutions to aggregate information and collaborate with a master model.
“Federated learning offers tremendous promise,” said Marius George Linguraru, D.Phil., M.A., M.Sc., the Connor Family Professor of Research and Innovation, principal investigator at the Sheikh Zayed Institute of Pediatric Surgical Innovation and senior author on the manuscript. “As a community of experts, we have found that federated learning allows us to move away from the challenges of sharing data in central repositories. Instead, we share the models, which can be designed to protect privacy by limiting what’s shared outside of any given institution.”
A champion of pediatric health, Linguraru wants to ensure that children are represented in the development of models that advance science and medicine. “Sharing data is even more crucial when there are few patients, such as in rare diseases or pediatric populations,” he said. “In general, healthcare data suffers from inequitable representation in our public health systems and services.”
Learn more here about the challenges and potential solutions from experts at Rhino Health, Johns Hopkins University School of Medicine, NVIDIA, University of Cambridge, Ben-Gurion University Israel, MD Anderson Cancer Center, Dana-Farber Cancer Institute and Children’s National Hospital.
AI team wins international competition to measure pediatric brain tumors
Children’s National Hospital scientists won first place in a global competition to use artificial intelligence (AI) to analyze pediatric brain tumor volumes, demonstrating the team’s ground-breaking advances in imaging and machine learning.
During the International Conference on Medical Image Computing and Computer Assisted Intervention (MICCAI), the Children’s National team demonstrated the most accurate algorithm to study the volume of brain tumors – the most common solid tumors affecting children and adolescents and a leading cause of disease-related death at this young age. The technology could someday help oncologists understand the extent of a patient’s disease, quantify the efficacy of treatments and predict patient outcomes.
“The Brain Tumor Segmentation Challenge inspires leaders in medical imaging and deep learning to try to solve some of the most vexing problems facing radiologists, oncologists, computer engineers and data scientists,” said Marius George Linguraru, D.Phil., M.A., M.Sc., the Connor Family Professor in Research and Innovation and principal investigator in the Sheikh Zayed Institute for Pediatric Surgical Innovation. “I am honored that our team won, and I’m even more thrilled for our clinicians and their patients, who need us to keep moving forward to find new ways to treat pediatric brain tumors.”
Why we’re excited
With roughly 4,000 children diagnosed yearly, pediatric brain tumors are consistently the most common type of pediatric solid tumor, second only to leukemia in pediatric malignancies. At the urging of Linguraru and one of his peers at the Children’s Hospital of Philadelphia, pediatric data was included in the international competition for the first time, helping to ensure that children are represented in medical and technological advances.
The contest required participants to use data from multiple institutions and consortia to test competing methods fairly. The Children’s National team created a method to tap into the power of two types of imaging and machine learning: 3D convolutional neural network and 3D Vision Transformer-based deep learning models. They identified regions of the brain affected by tumors, made shrewd data-processing decisions driven by the team’s experience in AI for pediatric healthcare and achieved state-of-the-art results.
The competition drew 18 teams who are leaders from across the AI and machine learning community. The runner-up teams were from NVIDIA and the University of Electronic Science and Technology of China.
The big picture
“Children’s National has an all-star lineup, and I am thrilled to see our scientists recognized on an international stage,” said interim Executive Vice President and Chief Academic Officer Catherine Bollard, M.D., M.B.Ch.B., director of the Center for Cancer for Immunology Research. “As we work to attack brain tumors from multiple angles, we continue to show our exceptional ability to create new and better tools for diagnosing, imaging and treating these devastating tumors.”
“Mask up!” Soon, AI may be prompting healthcare workers
Researchers at Children’s National Hospital are embarking on an effort to deploy computer vision and artificial intelligence (AI) to ensure medical professionals appropriately use personal protective equipment (PPE). This strikingly common problem touches almost every medical specialty and setting.
With nearly $2.2 million in grants from the National Institutes of Health, the team is combining their expertise with information scientists at Drexel University and engineers at Rutgers University to build a system that will alert doctors, nurses and other medical professionals of mistakes in how they are wearing their PPE. The goal is to better protect healthcare workers (HCWs) from dangerous viruses and bacteria that they may encounter — an issue laid bare with the COVID-19 pandemic and PPE shortages.
“If any kind of healthcare setting says they don’t have a problem with PPE non-adherence, it’s because they’re not monitoring it,” said Randall Burd, M.D., Ph.D., division chief of Trauma and Burn Surgery at Children’s National and the principal investigator on the project. “We need to solve this problem, so the medical community will be prepared for the next potential disaster that we might face.”
The big picture
The World Health Organization has estimated that between 80,000 and 180,000 HCWs died globally from COVID-19 between January 2020 and May 2021 — an irreplaceable loss of life that created significant gaps in the pandemic response. Research has shown that HCWs had an 11-fold greater infection risk than the workers in other professions, and those who were not wearing appropriate PPE had a 1/3 higher infection risk, compared to peers who followed best practices.
Burd said the Centers for Disease Control and Prevention has recommended that hospitals task observers to stand in the corner with a clipboard to watch clinicians work and confirm that they are being mindful of their PPE. However, “that’s just not scalable,” he said. “You can’t always have someone watching, especially when you may have 50 people in and out of an operating room on a challenging case. On top of that, the observers are generally trained clinicians who could be filling other roles.”
What’s ahead
Bringing together the engineering talents at Drexel and Rutgers with the clinical and machine-learning expertise at Children’s National, the researchers plan to build a computer-vision system that will watch whether HCWs are properly wearing PPE such as gloves, masks, eyewear, gowns and shoe covers.
The team is contemplating how the system will alert HCWs to any errors and is considering haptic watch alerts and other types of immediate feedback. The emerging power of AI brings tremendous advantages over the current, human-driven systems, said Marius George Linguraru, D.Phil., M.A., M.Sc., the Connor Family Professor in Research and Innovation at Children’s National and principal investigator in the Sheikh Zayed Institute for Pediatric Surgical Innovation.
“Human observers only have one pair of eyes and may fatigue or get distracted,” Linguraru said. “Yet artificial intelligence, and computers in general, work without getting tired. We are excited to figure out how a computer can do this work – without ever blinking.”
Children’s National Hospital leads the way
Linguraru says that Children’s National and its partners make up the ideal team to tackle this vexing challenge because of their ability to assemble a multidisciplinary team of scientists and engineers who can work together with clinicians. “This is a dialogue,” he said. “A computer scientist, like myself, needs to understand the intricacies of complicated clinical realities, while a clinician needs to understand how AI can impact the practice of medicine. The team we are bringing together is intentional and poised to fix this problem.”
Marius George Linguraru, D.Phil., M.A., M.Sc., named as Connor Family Professor of Research and Innovation
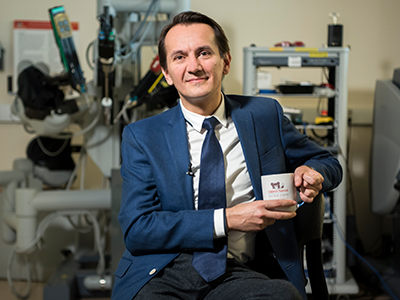
“Artificial Intelligence may be the greatest tool we have for improving the quality of and access to medical care for children, especially those most vulnerable to health system inequities,” said Dr. Linguraru. “This professorship will help me extend our leadership in this vital field. The tools and care strategies we develop will benefit children worldwide.”
Children’s National Hospital named Marius George Linguraru, D.Phil., M.A., M.Sc., as the Connor Family Professor of Research and Innovation at Children’s National Hospital.
Dr. Linguraru is a principal investigator in the Sheikh Zayed Institute for Pediatric Surgical Innovation at Children’s National. He directs the award-winning Precision Medicine Imaging Group. He is also a professor of Radiology and Pediatrics and secondary professor of Biomedical Engineering at George Washington University.
About the award
Dr. Linguraru joins a distinguished group of 42 Children’s National physicians and scientists who hold an endowed chair. Professorships at Children’s National support groundbreaking work on behalf of children and their families and foster new discoveries and innovations in pediatric medicine. These appointments carry prestige and honor that reflect the recipient’s achievements and donor’s forethought to advance and sustain knowledge.
Dr. Linguraru is a global leader in harnessing the power of quantitative imaging and machine learning to rapidly and positively impact children’s health. Dr. Linguraru and his team use artificial intelligence (AI) and digital technology innovations to improve access to healthcare and the understanding of rare and newborn diseases. Their work enables clinicians to deliver care faster, evaluate responses to treatments and prevent health complications. They have positioned Children’s National as an international leader in the development of pediatric AI to ensure equitable care for all children.
“Artificial Intelligence may be the greatest tool we have for improving the quality of and access to medical care for children, especially those most vulnerable to health system inequities,” said Dr. Linguraru. “This professorship will help me extend our leadership in this vital field. The tools and care strategies we develop will benefit children worldwide.”
About the donors
The Connor family, through their vision and generosity, are ensuring that Dr. Linguraru and future holders of this professorship will launch bold, new initiatives to rapidly advance the field of pediatric research and innovation, elevate our leadership and improve the lifetimes of children.
“We strongly believe in the power of academic entrepreneurship to improve the health and wellbeing of children,” said Ed and Chris Connor, who are longtime donors and members of the Children’s National community. “This endowment is our way of supporting Children’s National’s work in research and innovation and recognizing Dr. Linguraru’s international leadership in using AI to benefit child health.”
AI: The “single greatest tool” for improving access to pediatric healthcare
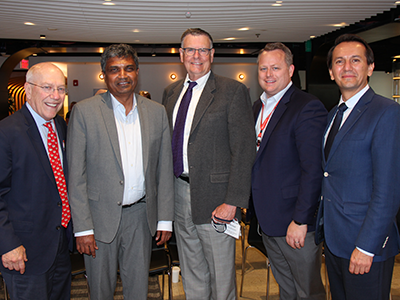
The daylong event drew experts from the Food and Drug Administration, Pfizer, Oracle Health, NVIDIA, AWS Health and elsewhere to start building a community aimed at using data for the advancement of pediatric medicine.
The future of pediatric medicine holds the promise of artificial intelligence (AI) that can help diagnose rare diseases, provide roadmaps for safer surgeries, tap into predictive technologies to guide individual treatment plans and shrink the distance between patients in rural areas and specialty care providers.
These and dozens of other innovations were contemplated as scientists came together at the inaugural symposium on AI in Pediatric Health and Rare Diseases, hosted by Children’s National Hospital and the Fralin Biomedical Research Institute at Virginia Tech. The daylong event drew experts from the Food and Drug Administration, Pfizer, Oracle Health, NVIDIA, AWS Health and elsewhere to start building a community aimed at using data for the advancement of pediatric medicine.
“AI is the single greatest tool for improving equity and access to health care,” said symposium host Marius George Linguraru, D.Phil., M.A., M.Sc., principal investigator at the Sheikh Zayed Institute for Pediatric Surgical Innovation. “As a population, kids are vastly underrepresented in scientific research and resulting treatments, but pediatric specialties can use AI to provide medical care to kids more efficiently, more quickly and more effectively.”
What they’re saying
Scientists shared their progress in building digital twins to predict surgical outcomes, enhancing visualization to increase the precision of delicate interventions, establishing data command centers to anticipate risks for fragile patients and more. Over two dozen speakers shared their vision for the future of medicine, augmented by the power of AI:
- Keynote speaker Subha Madhavan, Ph.D., vice president and head of AI and machine learning at Pfizer, discussed various use cases and the potential to bring drugs to market faster using real-world evidence and AI. She saw promise for pediatrics. “This is probably the most engaging mission: children’s health and rare diseases,” she said. “It’s hard to find another mission that’s as compelling.”
- Brandon J. Nelson, Ph.D., staff fellow in the Division of Imaging, Diagnostics and Software Reliability at the Food and Drug Administration, shared ways AI will improve diagnostic imaging and reduce radiation exposure to patients, using more advanced image reconstruction and denoising techniques. “That is really our key take-home message,” he said. “We can get what … appear as higher dose images, but with less dose.”
- Daniel Donoho, M.D., a neurosurgeon at Children’s National, introduced the audience to the potential of “Smart ORs”: operating rooms where systems can ingest surgery video and provide feedback and skill assessments. “We have to transform the art of surgery into a measurable and improvable scientific practice,” he said.
- Debra Regier, M.D., chief of Genetics and Metabolism at Children’s National, discussed how AI could be used to diagnose and treat rare diseases by conducting deep dives into genetics and studying dysmorphisms in patients’ faces. Already, Children’s National has designed an app – mGene – that measures facial features and provides a risk score to help anyone in general practice determine if a child has a genetic condition. “The untrained eye can stay the untrained eye, and the family can continue to have faith in their provider,” she said.
What’s next
Linguraru and others stressed the need to design AI for kids, rather than borrow it from adults, to ensure medicine meets their unique needs. He noted that scientists will need to solve challenges, such as the lack of data inherent in rare pediatric disorders and the simple fact that children grow. “Children are not mini-adults,” Linguraru said. “There are big changes in a child’s life.”
The landscape will require thoughtfulness. Naren Ramakrishnan, Ph.D., director of the Sanghani Center for Artificial Intelligence & Analytics at Virginia Tech and symposium co-host, said that scientists are heading into an era with a new incarnation of public-private partnerships, but many questions remain about how data will be shared and organizations will connect. “It is not going to be business as usual, but what is this new business?” he asked.
$1.6m grant to boost MRI access globally for maternal, child health
Researchers at Children’s National Hospital are investigating ways to bring more portable and accessible low-field magnetic resonance imaging (MRI) to parts of the world that lack access to this critical diagnostic tool, thanks to a grant from the Bill & Melinda Gates Foundation.
The nearly $1.6 million in funding will enable clinicians to better treat pediatric neurological conditions including ischemic brain injury, hydrocephalus, micro- and macrocephaly and more, using analysis tools that are designed to handle the loss in image quality and related challenges inherent to low-field MRI. The research brings together teams at Children’s National and Children’s Hospital Los Angeles — two organizations with extensive experience in designing processing software tools for pediatric brain MRI analysis and data enhancement.
The patient benefit
“For 30 years, MRI has primarily helped patients in high-income countries. Our team is thrilled by the prospect of expanding this powerful tool to patients coming from a wide range of nations, geographies and socioeconomic backgrounds,” said Marius George Linguraru, D.Phil., M.A., M.Sc., principal investigator at the Sheikh Zayed Institute for Pediatric Surgical Innovation (SZI). “Low-field MRI comes with great advantages including portability at the point of care of patients, lower clinical costs and the elimination of sedation for young children.”
Linguraru and his long-time collaborator, Natasha Lepore, Ph.D., principal investigator at The Saban Research Institute at Children’s Hospital Los Angeles, will analyze data from the brains of children from birth for the maternal and child health studies. The MRI data analyzed will form the basis for future studies of children’s brain anatomy in health and disease.
The big picture
Through the new grant, researchers will develop a suite of tools to help clinicians better analyze data and images from low-field MRI systems. These systems already have been integrated into interventional and observational studies to help characterize early neurodevelopmental patterns and identify drivers of abnormal development. They are also evaluating the efficacy of maternal and infant-focused interventions aimed at improving neurodevelopmental outcomes.
Why we’re excited
At Children’s National, SZI has installed a Hyperfine Swoop system, and Linguraru’s team is creating image enhancement tools tailored to the unique challenges of low-field MRI. Chief among them, conventional processing tools developed over the past several decades remain incompatible with the low-field data and require new software to take full advantage of the diagnostic power of imaging.
The work brings together a prestigious international consortium of scientists and clinicians from around the world to harness the power of computing and expand the reach of diagnostic imaging. Lepore said the team is eager to bring modern medical imaging to parts of the world that have missed its many benefits.
“Children’s brain development in underserved areas can be affected by so many factors, like malnutrition or anemia,” Lepore said. “The software we will design for the Hyperfine scanners will improve research into these factors, so the optimal interventions can be designed. We are excited to bring our expertise to this important and timely project.”
With COVID-19, artificial intelligence performs well to study diseased lungs
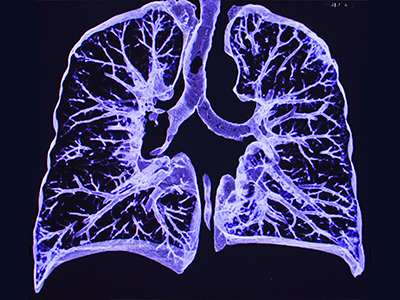
New research shows that artificial intelligence can be rapidly designed to study the lung images of COVID-19 patients.
Artificial intelligence can be rapidly designed to study the lung images of COVID-19 patients, opening the door to the development of platforms that can provide more timely and patient-specific medical interventions during outbreaks, according to research published this month in Medical Image Analysis.
The findings come as part of a global test of AI’s power, called the COVID-19 Lung CT Lesion Segmentation Challenge 2020. More than 2,000 international teams came together to train the power of machine learning and imaging on COVID-19, led by researchers at Children’s National Hospital, AI tech giant NVIDIA and the National Institutes of Health (NIH).
The bottom line
Many of the competing AI platforms were successfully trained to analyze lung lesions in COVID-19 patients and measure acute issues including lung thickening, effusions and other clinical findings. Ten leaders were named in the competition, which ran between November and December 2020. The datasets included patients with a range of ages and disease severity.
Yet work remains before AI could be implemented in a clinical setting. The AI models performed comparably to radiologists when analyzing data similar to what the algorithms had already encountered. However, the AI was less valuable when trained on fresh data from other sources during the testing phase, indicating that systems may need to study larger and more diverse data sets to meet their full potential. This is a challenge with AI that has been noted by others too.
What they’re saying
“These are the first steps in learning how we can quickly and accurately train AI for clinical use,” said Marius George Linguraru, D.Phil., M.A., M.Sc., principal investigator at the Sheikh Zayed Institute for Pediatric Surgical Innovation at Children’s National, who led the Grand Challenge Initiative. “The global interest in COVID-19 gave us a groundbreaking opportunity to address a health crisis, and multidisciplinary teams can now focus that interest and energy on developing better tools and methods.”
Holger Roth, senior applied research scientist at NVIDIA, said the challenge gave researchers around the world a shared platform for developing and evaluating AI algorithms to quickly detect and quantify COVID lesions from lung CT images. “These models help researchers visualize and measure COVID-specific lesions of infected patients and can facilitate timelier and patient-specific medical interventions to better treat COVID,” he said.
Moving the field forward
The organizers see great potential for clinical use. In areas with limited resources, AI could help triage patients, guide the use of therapeutics or provide diagnoses when expensive testing is unavailable. AI-defined standardization in clinical trials could also uniformly measure the effects of the countermeasures used against the disease.
Linguraru and his colleagues recommend more challenges, like the lung segmentation challenge, to develop AI applications in biomedical spaces that can test the functionality of these platforms and harness their potential. Open-source AI algorithms and public curated data, such as those offered through the COVID-19 Lung CT Lesion Segmentation Challenge 2020, are valuable resources for the scientific and clinical communities to work together on advancing healthcare.
“The optimal treatment of COVID-19 and other diseases hinges on the ability of clinicians to understand disease throughout populations – in both adults and children,” Linguraru said. “We are making significant progress with AI, but we must walk before we can run.”
Integrating clinical parameters with lung imaging to predict respiratory outcomes in premature babies
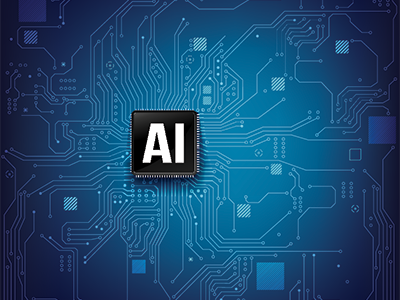
The team will develop an objective framework to predict the risk and assess the severity of respiratory disease in premature babies using non-invasive low-radiation X-ray imaging biomarkers and clinical parameters from the patient bedside.
Children’s National Hospital received a $1.7M award from the National Institutes of Health (NIH) National Heart, Lung, and Blood Institute (NHLBI) to develop computational tools that integrate continuous clinical parameters with lung imaging to predict respiratory outcomes for babies born severely premature in newborn intensive care unit (NICU) settings.
The multi-disciplinary team of internationally recognized experts in quantitative imaging, machine learning and neonatal respiratory research believes they can improve clinical practice. To get there, they will develop an objective framework to predict the risk and assess the severity of respiratory disease in premature babies using non-invasive low-radiation X-ray imaging biomarkers and clinical parameters from the patient bedside.
“This computational tool will assist clinicians in making critical decisions about the course of therapy and other necessary follow-ups,” said Gustavo Nino, M.D., M.S.H.S., D’A.B.S.M., principal investigator in the Center for Genetic Medicine at Children’s National. “An objective informed decision about the severity of lung disease in prematurity will result in fewer rehospitalizations, better long-term outcomes and life-saving benefits.”
Prematurity is the largest single cause of death in children under five in the world. Lower respiratory tract infections (LRTI) are the top cause of hospitalization and mortality in premature infants. Clinical tools to predict the risk and assess the severity of LRTI in premature babies are needed to allow early interventions that can decrease the high morbidity and mortality in this patient group.
“Our new technology will provide clinicians an accurate, fast and comprehensive summary of the respiratory status of premature babies,” said Dr. Nino. “The data analysis along with the software technology will help determine if a premature baby seen in the NICU can be safely discharged or will require further monitoring and treatment.”
Predictive analytics could help in many ways. For example, there are instances where newborns in the NICU are on the right path with no risks in the future, but there are babies who will come back with severe infections.
“In the first scenario, if we can predict earlier that they’re fine, this could reduce the number of chest X-rays and extra tests, so we assess that this child can be safely sent home,” said Marius George Linguraru, D.Phil., M.A., M.Sc., principal investigator in the Sheikh Zayed Institute for Pediatric Surgical Innovation at Children’s National. “On the other hand, for kids that may come back to the hospital in the near future, we could predict earlier that they are not that well by looking at images and other continuous measurements such as supplemental oxygen.”
This approach, in essence, is a collection of continuous data from the NICU, which is very complex itself because it needs to be collected every day and fed into a machine learning model that digests the data to identify risk patterns for the health of the lung.
“If we find that there is still a risk, it does not necessarily mean that the child has to stay in the NICU any longer, but they might continue treatment, and we will have to define how this integrates into the clinical management of these patients,” said Linguraru. “If there is something in the data that we can put our finger on, we will know which kids require timely attention, hopefully reducing future adverse situations with potential comorbidities and financial burdens.”
How radiologists and data scientists can collaborate to advance AI in clinical practice
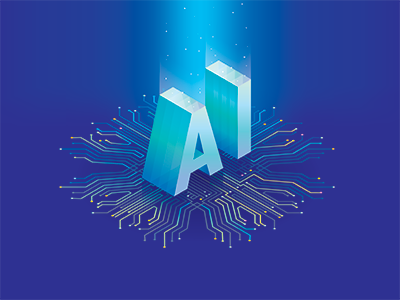
The scientific community continues to debate AI’s possibility of outperforming humans in specific tasks. In the context of the machine’s performance versus the clinician, Linguraru et al. argue that the community must consider social, psychological and economic contexts in addition to the medical implications to answer this puzzling question.
In a special report published in Radiology: Artificial Intelligence, a Children’s National Hospital expert and other institutions discussed a shared multidisciplinary vision to develop radiologic and medical imaging techniques through advanced quantitative imaging biomarkers and artificial intelligence (AI).
“AI algorithms can construct, reconstruct and interpret radiologic images, but they also have the potential to guide the scanner and optimize its parameters,” said Marius George Linguraru, D.Phil., M.A., M.Sc., principal investigator in the Sheikh Zayed Institute for Pediatric Surgical Innovation at Children’s National. “The acquisition and analysis of radiologic images is personalized, and radiologists and technologists adapt their approach to every patient based on their experience. AI can simplify this process and make it faster.”
The scientific community continues to debate AI’s possibility of outperforming humans in specific tasks. In the context of the machine’s performance versus the clinician, Linguraru et al. argue that the community must consider social, psychological and economic contexts in addition to the medical implications to answer this puzzling question.
Still, they believe that developing a useful radiologic AI system designed with the participation of radiologists could complement and possibly surpass human’s interpretation of the visuals.
Given AI’s potential applications, the authors encouraged radiologists to access many freely available resources to learn about machine learning, and radiomics to familiarize with basic concepts. Coursera, for example, can teach radiologists about convolutional neural networks and other techniques used by AI researchers.
Conversely, AI experts must reach out to radiologists and participate in public speaking events about their work. According to the researchers, during those engagement opportunities, clinicians understood the labor-saving benefits of automatic complex measurements on millions of images—something that they have been doing manually for years.
There are also hurdles around this quest of automation, which Linguraru et al. hope both fields can sort out by working together. A critical challenge that the experts mentioned was earning the trust of clinicians that are skeptical about the “black box” functionality of AI models, which makes it hard to understand and explain the behavior of a model.
Some questions, too, need answers on how to best leverage both human intelligence and AI by using human-in-the-loop where people train, tune, and test a particular algorithm, or AI in-the-loop where this different framing generates AI input and reflection in human systems.
“The key is to have a good scientific premise to adequately train and validate the algorithms and make them clinically useful. At that point, we can trust the box,” said Linguraru. “In radiology, we should focus on AI systems with radiologists in-the-loop, but also on training radiologists with AI in-the-loop, particularly as AI systems are getting smarter and learning to work better with radiologists.”
The experts also provided possible solutions to sharing large datasets, how to build datasets that allows robust investigations and how to improve the quality of a model that might be compared against human’s gold standard.
This special report is the second in a series of panel discussions hosted by the Radiological Society of North America and the Medical Image Computing and Computer Assisted Intervention Society. The discussion builds upon the first in the series “Machine Learning for Radiology from Challenges to Clinical Applications” that touched on how to incentivize annotators to participate in projects, the promotion of “team science” to address research questions and challenges, among other topics.
Researchers awarded $3.5 million to study brain and cranium development in children
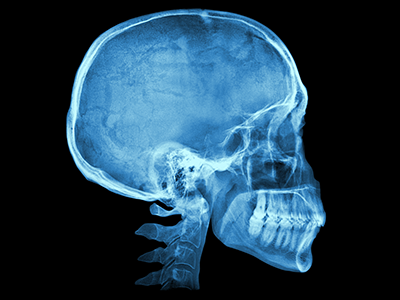
Currently, studies on typical brain and cranium development are limited. One reason for this is that imaging techniques are optimized to best visualize either bone or soft tissue, but not both.
With prevalence of developmental disorders on the rise, the need to understand brain development has never been more critical. Development of the brain is strongly influenced by the cranium, but this relationship has not been adequately studied because of limitations in imaging technology. Now, researchers from Children’s Hospital Los Angeles and Children’s National Hospital are working together to develop techniques that will provide greater insight into this relationship. Their studies will be funded by The National Institute of Dental and Craniofacial Research, which has awarded them $3.5 million.
Natasha Leporé, Ph.D., of Children’s Hospital Los Angeles, studies methods to interpret brain imaging data. “There’s a lot of interaction between the skull and the brain,” she says, “and we want to better understand how they grow together.”
Currently, studies on typical brain and cranium development are limited. One reason for this is that imaging techniques are optimized to best visualize either bone or soft tissue, but not both.
The brain — mostly composed of water, protein and fat — doesn’t show up well on computerized tomography (CT) scans, which use X-ray images. In addition, radiation exposure limits the amount of CT scan data available in children. On the other hand, magnetic resonance imaging (MRI) scans are excellent for brain images but are not optimal for surrounding bone.
This presents researchers with a dilemma if they want to see the brain and the skull together in one image. Fortunately, research barriers like these are often overcome by collaboration.
Leporé will work with Marius George Linguraru, D.Phil, M.A., MS.c., principal investigator in the Sheikh Zayed Institute for Pediatric Surgical Innovation at Children’s National Hospital.
Linguraru works on a set of tools for cranial phenotyping, using existing CT images from typically developing children. In their collaboration, Leporé and Linguraru will extend the tools to MRI scans, allowing the team to analyze the brain and cranium simultaneously. The pair has received a $3.5 million award over 5 years.
“The tools we develop together will help us to better understand the healthy growth of children,” says Linguraru. “We will have the ability to analyze the joint cranial and brain development from large medical image datasets of pediatric patients.”
This, the team says, will be invaluable to the medical community.
“These tools will help clinicians to better assess, diagnose and plan treatment for infants with cranial deformities,” says Linguraru.
Collaborations like this allow expertise to be shared across specialties, ultimately benefiting children in need. Exceptional pediatric care is a result of teamwork; not only doctors, nurses and clinical staff, but also biomedical research, which arms clinicians with the information they depend on.
“We need to have a clear idea of what is expected in normal development,” says Leporé. “This allows doctors to detect and better understand differences in development.”
Other members of the research team include: Vidya Rajagopalan, Ph.D.; Marvin Nelson, M.D.; Alexis Johns, Ph.D.; Niharika Gajawelli, Ph.D. (from Children’s Hospital Los Angeles and University of Southern California); Robert Keating, M.D. (Children’s National Hospital); Yalin Wang, Ph.D. (Arizona State University); Antonio Porras, Ph.D. (University of Colorado); Sean Deoni, Ph.D. (Rhode Island Hospital and Brown University).
A version of this story appeared on the Children’s Hospital Los Angeles newsroom.
Chest X-rays help distinguish COVID-19 from other types of viral respiratory infections
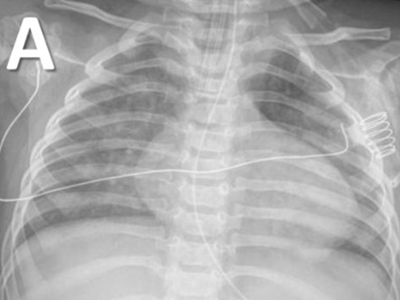
COVID-19 in a 9-month infant demonstrating a GGO/consolidation pattern.
Increased infections of COVID-19 and other respiratory viruses in kids are filling up children’s hospitals, pushing them to critical occupancy nationwide. As schools open, the community spread of viral infections has become more common, and the rapid differentiation of pediatric COVID-19 from other viruses is — more than ever — relevant to pediatric clinicians.
“Pediatric cases have increased exponentially and currently represent over 15% of the total cases, and about 26% of the new infections in the U.S. Chest imaging is a powerful tool for determining their status.” said Marius George Linguraru, D.Phil., M.A., M.Sc., principal investigator in the Sheikh Zayed Institute for Pediatric Surgical Innovation at Children’s National Hospital.
In a new peer-reviewed study, researchers from Children’s National found novel and clinically relevant data regarding the specific lung imaging patterns of pediatric COVID-19 on chest radiographs (CXR), their relationship to clinical outcomes and the possible differences from infections caused by other respiratory viruses in children.
“While most studies have focused on clinical manifestations and lung imaging of COVID-19 in adults, this study is the first to define specific patterns of clinical disease and imaging signatures in CXR in different age groups of children infected with COVID-19,” said Gustavo Nino, M.D., director of sleep medicine at Children’s National.
Lung imaging has become critically important for the early identification and treatment of pediatric patients affected by COVID-19 and may play an important role in distinguishing COVID-19 infection from viral bronchiolitis.
“The old perception that COVID-19 only affects older patients is no longer true,” said Dr. Nino. “Pediatric intensive care units and emergency departments are overwhelmed with COVID-19 cases, and now hospitals are admitting more children with COVID-19 than ever.”
As next steps, Nino et al. will develop pediatric-centered technology for early identification, risk stratification, and outcome prediction of COVID-19 in children, similar to what the scientific community has done for adults.
Machine learning tool detects the risk of genetic syndromes
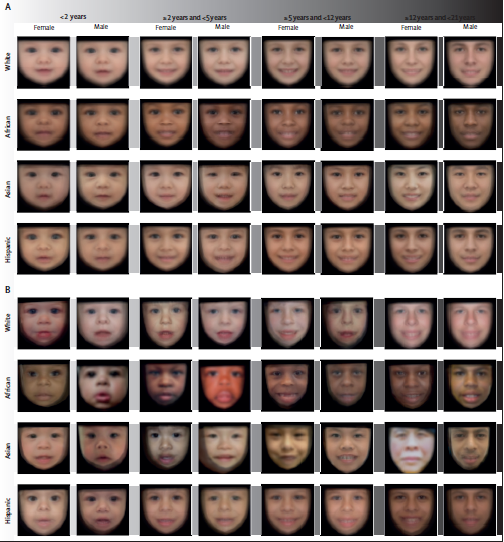
(A) Control population. (B) Population with Williams-Beuren syndrome. Average faces were generated for each demographic group after automatic face pose correction.
With an average accuracy of 88%, a deep learning technology offers rapid genetic screening that could accelerate the diagnosis of genetic syndromes, recommending further investigation or referral to a specialist in seconds, according to a study published in The Lancet Digital Health. Trained with data from 2,800 pediatric patients from 28 countries, the technology also considers the face variability related to sex, age, racial and ethnic background, according to the study led by Children’s National Hospital researchers.
“We built a software device to increase access to care and a machine learning technology to identify the disease patterns not immediately obvious to the human eye or intuition, and to help physicians non-specialized in genetics,” said Marius George Linguraru, D.Phil., M.A., M.Sc., principal investigator in the Sheikh Zayed Institute for Pediatric Surgical Innovation at Children’s National Hospital and senior author of the study. “This technological innovation can help children without access to specialized clinics, which are unavailable in most of the world. Ultimately, it can help reduce health inequality in under-resourced societies.”
This machine learning technology indicates the presence of a genetic syndrome from a facial photograph captured at the point-of-care, such as in pediatrician offices, maternity wards and general practitioner clinics.
“Unlike other technologies, the strength of this program is distinguishing ‘normal’ from ‘not-normal,’ which makes it an effective screening tool in the hands of community caregivers,” said Marshall L. Summar, M.D., director of the Rare Disease Institute at Children’s National. “This can substantially accelerate the time to diagnosis by providing a robust indicator for patients that need further workup. This first step is often the greatest barrier to moving towards a diagnosis. Once a patient is in the workup system, then the likelihood of diagnosis (by many means) is significantly increased.”
Every year, millions of children are born with genetic disorders — including Down syndrome, a condition in which a child is born with an extra copy of their 21st chromosome causing developmental delays and disabilities, Williams-Beuren syndrome, a rare multisystem condition caused by a submicroscopic deletion from a region of chromosome 7, and Noonan syndrome, a genetic disorder caused by a faulty gene that prevents normal development in various parts of the body.
Most children with genetic syndromes live in regions with limited resources and access to genetic services. The genetic screening may come with a hefty price tag. There are also insufficient specialists to help identify genetic syndromes early in life when preventive care can save lives, especially in areas of low income, limited resources and isolated communities.
“The presented technology can assist pediatricians, neonatologists and family physicians in the routine or remote evaluation of pediatric patients, especially in areas with limited access to specialized care,” said Porras et al. “Our technology may be a step forward for the democratization of health resources for genetic screening.”
The researchers trained the technology using 2,800 retrospective facial photographs of children, with or without a genetic syndrome, from 28 countries, such as Argentina, Australia, Brazil, China, France, Morocco, Nigeria, Paraguay, Thailand and the U.S. The deep learning architecture was designed to account for the normal variations in the face appearance among populations from diverse demographic groups.
“Facial appearance is influenced by the race and ethnicity of the patients. The large variety of conditions and the diversity of populations are impacting the early identification of these conditions due to the lack of data that can serve as a point of reference,” said Linguraru. “Racial and ethnic disparities still exist in genetic syndrome survival even in some of the most common and best-studied conditions.”
Like all machine learning tools, they are trained with the available dataset. The researchers expect that as more data from underrepresented groups becomes available, they will adapt the model to localize phenotypical variations within more specific demographic groups.
In addition to being an accessible tool that could be used in telehealth services to assess genetic risk, there are other potentials for this technology.
“I am also excited about the potential of the technology in newborn screening,” said Linguraru. “There are approximately 140 million newborns every year worldwide of which eight million are born with a serious birth defect of genetic or partially genetic origin, many of which are discovered late.”
Children’s National as well recently announced that it has entered into a licensing agreement with MGeneRx Inc. for its patented pediatric medical device technology. MGeneRx is a spinoff from BreakThrough BioAssets LLC, a life sciences technology operating company focused on accelerating and commercializing new innovations, such as this technology, with an emphasis on positive social impact.
“The social impact of this technology cannot be underestimated,” said Nasser Hassan, acting chief executive officer of MGeneRx Inc. “We are excited about this licensing agreement with Children’s National Hospital and the opportunity to enhance this technology and expand its application to populations where precision medicine and the earliest possible interventions are sorely needed in order to save and improve children’s lives.”
Commercialization of novel facial analysis technology can improve diagnosis of rare disorders in pediatric patients
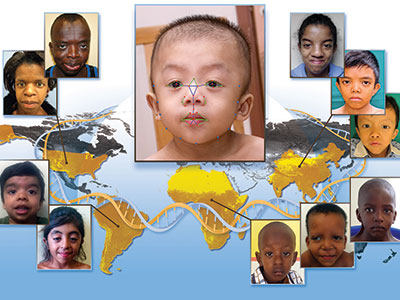
Children’s National Hospital has entered into a licensing agreement with MGeneRx Inc. for its patented pediatric medical device technology using objective digital biometric analysis software for the early and non-invasive screening of dysmorphic genetic diseases such as Noonan syndrome.
Children’s National Hospital has entered into a licensing agreement with life sciences technology company MGeneRx Inc. for its patented pediatric medical device technology using objective digital biometric analysis software for the early and non-invasive screening of dysmorphic genetic diseases. The technology, developed by a multidisciplinary Children’s National team led by Marius George Linguraru, D.Phil, M.A., M.Sc., of the Sheikh Zayed Institute for Pediatric Surgical Innovation and Marshall Summar, M.D., director of the Children’s National Rare Disease Institute (CNRDI), can provide a more advanced diagnostic tool for regions of the world with limited access to geneticists or genetic testing.
The application utilizes artificial intelligence (AI) and machine learning to analyze biometric data and identify facial markers that are indicative of genetic disorders. Physicians can capture biometric data points of a child’s face in real time within the platform, where it scans facial biometric features to determine the potential presence of a genetic disease, which can often be life-threatening without early intervention. Research studies conducted in conjunction with the National Human Genome Research Institute at the National Institutes of Health further enhanced the development of the application in recent years, showing the potential to detect, with a 90 percent accuracy, early diagnosis of 128 genetic diseases across pediatric subjects in 28 countries. These diseases include DiGeorge syndrome (22q11.2 deletion syndrome), Down syndrome, Noonan syndrome and Williams-Beuren syndrome.
“We are delighted to enter into this licensing agreement through Innovation Ventures, the commercialization arm of Children’s National Hospital, which seeks to move inventions and discoveries from Children’s National to the marketplace to benefit the health and well-being of children. Our mission is to add the ‘D’ in development to the ‘R’ in research to accelerate the commercialization of our intellectual property,” says Kolaleh Eskandanian, Ph.D., M.B.A., P.M.P., vice president and chief innovation officer at Children’s National and managing director of Innovation Ventures. “It is through partnerships with startups and the industry that we can achieve this goal and thus we highly value this new partnership with MGeneRx Inc. The acceleration and commercialization of this objective digital biometric analysis technology will not only help diagnose rare genetic disorders – it will also allow for earlier interventions that improve the quality of life for the children living with these conditions.”
Eskandanian adds that the social impact of this technology is especially profound in lower income nations around the world, where there is a high prevalence of rare genetic conditions but a severe lack in the specialty care required to diagnose and treat them. Additional data collected through the expanded use of the technology will help to further develop the application and expand its capabilities to identify and diagnose additional rare genetic conditions.
The licensing agreement was arranged by the Children’s National Office of Innovation Ventures, which is focused on the commercialization of impactful new pediatric medical device technologies and therapies to advance children’s health care. Created to catalyze the ongoing translational research of the Children’s National Research Institute (CNRI) as well as inventions by hospital’s clinicians, Innovation Ventures focuses on four core pillars to advance pediatric medical technologies including a Biodesign program, partnerships and alliances to augment internal capacity, seed funding to de-risk technologies and validate market and clinical relevance, and back-office operations to manage intellectual property and licensing activities. Since 2017, Children’s National intellectual property has served as the basis for over 15 licensing or option agreements with commercial partners.
Providing access to an array of experts and resources for pediatric innovators is one of the aims of the Children’s National Research & Innovation Campus, a first-of-its-kind focused on pediatric health care innovation, with the first phase currently open on the former Walter Reed Army Medical Center campus in Washington, D.C. With its proximity to federal research institutions and agencies, universities, academic research centers, as well as on-site incubator Johnson and Johnson Innovation – JLABS, the campus provides a rich ecosystem of public and private partners, which will help bolster pediatric innovation and commercialization.