Integrating clinical parameters with lung imaging to predict respiratory outcomes in premature babies
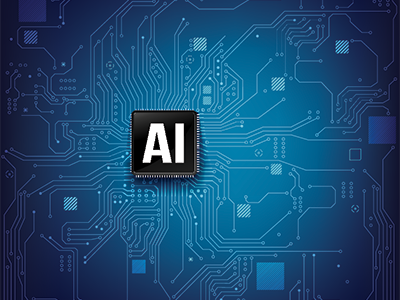
The team will develop an objective framework to predict the risk and assess the severity of respiratory disease in premature babies using non-invasive low-radiation X-ray imaging biomarkers and clinical parameters from the patient bedside.
Children’s National Hospital received a $1.7M award from the National Institutes of Health (NIH) National Heart, Lung, and Blood Institute (NHLBI) to develop computational tools that integrate continuous clinical parameters with lung imaging to predict respiratory outcomes for babies born severely premature in newborn intensive care unit (NICU) settings.
The multi-disciplinary team of internationally recognized experts in quantitative imaging, machine learning and neonatal respiratory research believes they can improve clinical practice. To get there, they will develop an objective framework to predict the risk and assess the severity of respiratory disease in premature babies using non-invasive low-radiation X-ray imaging biomarkers and clinical parameters from the patient bedside.
“This computational tool will assist clinicians in making critical decisions about the course of therapy and other necessary follow-ups,” said Gustavo Nino, M.D., M.S.H.S., D’A.B.S.M., principal investigator in the Center for Genetic Medicine at Children’s National. “An objective informed decision about the severity of lung disease in prematurity will result in fewer rehospitalizations, better long-term outcomes and life-saving benefits.”
Prematurity is the largest single cause of death in children under five in the world. Lower respiratory tract infections (LRTI) are the top cause of hospitalization and mortality in premature infants. Clinical tools to predict the risk and assess the severity of LRTI in premature babies are needed to allow early interventions that can decrease the high morbidity and mortality in this patient group.
“Our new technology will provide clinicians an accurate, fast and comprehensive summary of the respiratory status of premature babies,” said Dr. Nino. “The data analysis along with the software technology will help determine if a premature baby seen in the NICU can be safely discharged or will require further monitoring and treatment.”
Predictive analytics could help in many ways. For example, there are instances where newborns in the NICU are on the right path with no risks in the future, but there are babies who will come back with severe infections.
“In the first scenario, if we can predict earlier that they’re fine, this could reduce the number of chest X-rays and extra tests, so we assess that this child can be safely sent home,” said Marius George Linguraru, D.Phil., M.A., M.Sc., principal investigator in the Sheikh Zayed Institute for Pediatric Surgical Innovation at Children’s National. “On the other hand, for kids that may come back to the hospital in the near future, we could predict earlier that they are not that well by looking at images and other continuous measurements such as supplemental oxygen.”
This approach, in essence, is a collection of continuous data from the NICU, which is very complex itself because it needs to be collected every day and fed into a machine learning model that digests the data to identify risk patterns for the health of the lung.
“If we find that there is still a risk, it does not necessarily mean that the child has to stay in the NICU any longer, but they might continue treatment, and we will have to define how this integrates into the clinical management of these patients,” said Linguraru. “If there is something in the data that we can put our finger on, we will know which kids require timely attention, hopefully reducing future adverse situations with potential comorbidities and financial burdens.”