How radiologists and data scientists can collaborate to advance AI in clinical practice
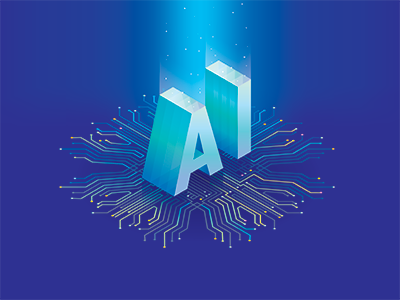
The scientific community continues to debate AI’s possibility of outperforming humans in specific tasks. In the context of the machine’s performance versus the clinician, Linguraru et al. argue that the community must consider social, psychological and economic contexts in addition to the medical implications to answer this puzzling question.
In a special report published in Radiology: Artificial Intelligence, a Children’s National Hospital expert and other institutions discussed a shared multidisciplinary vision to develop radiologic and medical imaging techniques through advanced quantitative imaging biomarkers and artificial intelligence (AI).
“AI algorithms can construct, reconstruct and interpret radiologic images, but they also have the potential to guide the scanner and optimize its parameters,” said Marius George Linguraru, D.Phil., M.A., M.Sc., principal investigator in the Sheikh Zayed Institute for Pediatric Surgical Innovation at Children’s National. “The acquisition and analysis of radiologic images is personalized, and radiologists and technologists adapt their approach to every patient based on their experience. AI can simplify this process and make it faster.”
The scientific community continues to debate AI’s possibility of outperforming humans in specific tasks. In the context of the machine’s performance versus the clinician, Linguraru et al. argue that the community must consider social, psychological and economic contexts in addition to the medical implications to answer this puzzling question.
Still, they believe that developing a useful radiologic AI system designed with the participation of radiologists could complement and possibly surpass human’s interpretation of the visuals.
Given AI’s potential applications, the authors encouraged radiologists to access many freely available resources to learn about machine learning, and radiomics to familiarize with basic concepts. Coursera, for example, can teach radiologists about convolutional neural networks and other techniques used by AI researchers.
Conversely, AI experts must reach out to radiologists and participate in public speaking events about their work. According to the researchers, during those engagement opportunities, clinicians understood the labor-saving benefits of automatic complex measurements on millions of images—something that they have been doing manually for years.
There are also hurdles around this quest of automation, which Linguraru et al. hope both fields can sort out by working together. A critical challenge that the experts mentioned was earning the trust of clinicians that are skeptical about the “black box” functionality of AI models, which makes it hard to understand and explain the behavior of a model.
Some questions, too, need answers on how to best leverage both human intelligence and AI by using human-in-the-loop where people train, tune, and test a particular algorithm, or AI in-the-loop where this different framing generates AI input and reflection in human systems.
“The key is to have a good scientific premise to adequately train and validate the algorithms and make them clinically useful. At that point, we can trust the box,” said Linguraru. “In radiology, we should focus on AI systems with radiologists in-the-loop, but also on training radiologists with AI in-the-loop, particularly as AI systems are getting smarter and learning to work better with radiologists.”
The experts also provided possible solutions to sharing large datasets, how to build datasets that allows robust investigations and how to improve the quality of a model that might be compared against human’s gold standard.
This special report is the second in a series of panel discussions hosted by the Radiological Society of North America and the Medical Image Computing and Computer Assisted Intervention Society. The discussion builds upon the first in the series “Machine Learning for Radiology from Challenges to Clinical Applications” that touched on how to incentivize annotators to participate in projects, the promotion of “team science” to address research questions and challenges, among other topics.